Table of Contents
Let’s have a glimpse of discrete probability distribution and its types. The main objective of this article is to understand the types of probability distribution and their examples. More focus will be on discrete probability distribution and its examples to understand it properly.
The probability distribution is a statistical term. It helps understand statistical concepts like confidence intervals and hypothesis testing. There are two types of probability distributions. However, we will focus only on the discrete probability distribution.
Before moving further, let me ask you a question. What is a discrete probability distribution? If you know the answer to this question, that’s good. Don’t worry if you are new to the above question. So, let’s discuss the discrete probability distribution.
What is a Discrete Probability Distribution?
We know that discrete probability distribution is a statistical term. It is a type of probability distribution. We can define it as “a term composed of discrete random variables.” If we explain it, we can say that the finite chances of occurrence of some variable. Here the variable should be discrete.
Now you might be wondering about what is a discrete random variable. The discrete random variable has a countable probability. It can be any finite numeric digit. Moreover, a discrete random variable is written chiefly in tabular form.
Discrete Probability Distribution Examples
Examples are the best way to understand something. Here we are giving an example of the discrete probability distribution. We hope that you might understand it better.
Let’s suppose we toss a coin. When it falls to the ground, what are the possible results? Of course, only two, and we have equal chances of getting any side. It might be heads or tails. So when you have a finite number of results for a probability distribution, it is known as discrete.
We can take another example while playing Ludo. When you roll dice with six sides, what is the possible result? You may get any one of the six sides, so there are only six possible results. Here also, we have equal chances of getting any side of the dice.
Types of Probability Distributions
Now comes the types of discrete probability distributions. We have two main types of probability distributions, and there are many subtypes in them. The two types of probability distribution are discrete and continuous probability distribution.
Discrete Probability Distributions
As we have already discussed this topic, we will now go for discrete probability distribution examples in statistics. Six prominent examples are Binomial Distribution, Hypergeometric Distribution, Geometric Distribution, Negative Binomial Distribution, Multinomial Distribution, and Poisson Distributions.
To understand these examples in a better way, let’s go through their definitions and a brief explanation. Binomial Distribution has two possibilities like success or failure. Bi means two. So, any experiment or survey that gives two possibilities even after multiple tries is binomial Distribution.
The number of failures you get in Bernoulli trials before getting success is named Geometric Distribution. The formula for finding a geometric distribution is f(x) = (1 − p)x − 1p. The probability density function f(x) represents it.
Geometric distribution differs from the binomial distribution. It is because the number of failures before the first success is counted. While in a binomial distribution, we have fixed numbers of experiments to be done. After that, we determine the possibility of success or failure.
When we talk about hypergeometric distributions, it is almost the same as a binomial distribution. The hypergeometric distribution is the probability of success when the size of the population remains the same during the trial.
A multinomial distribution has two or more possibilities. It helps to calculate the results of trials with more variables. The difference is binomial distribution has only two possibilities; heads or tails or success and failure.
Negative Binomial distribution occurs when we perform trials with only two variables. The number of trials we did before getting a fixed number of failures is a negative binomial distribution. It is almost opposite to the geometric distribution.
In Poisson distribution, we measure the number of trials during a specific time. It is named after the French mathematician Denis Poisson. As time is finite, the variable can have some specific values only.
Continuous Probability Distribution
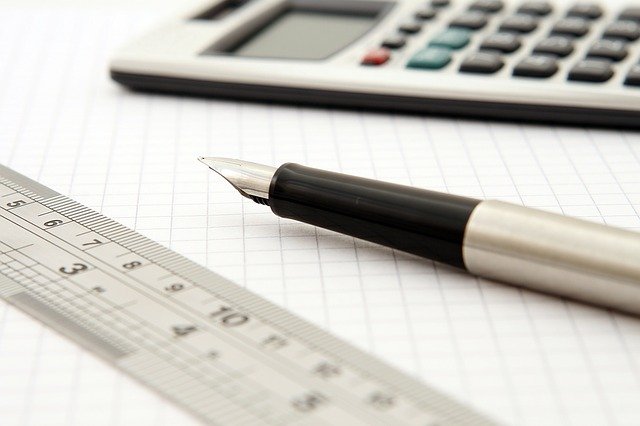
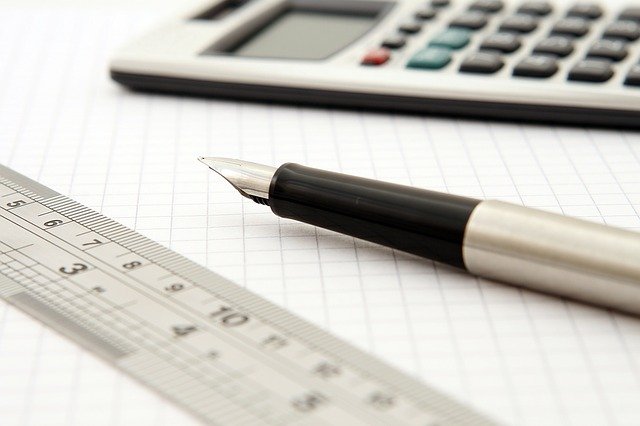
A continuous probability distribution is the opposite of discrete probability distribution. Here, the variables are unlimited, and the probabilities are also infinite. If we take the example of time, you can not guess it because time is unlimited.
A discrete probability distribution has some finite probabilities. When we toss a coin, we have two probabilities, heads and tails. But when we want to guess the weight of a man, we can not guess it. His weight may be 60.1kg or any digit that we can’t even guess, like 65.00172 kg.
As the discrete probability distribution has several types of examples, so does continuous probability distribution have. Beta distribution, Cauchy distribution, Exponential distribution, Gamma distribution, Logistic distribution, and Weibull distribution are the types of the continuous probability distribution.
The beta distribution shows the results of an experiment in proportions or percentages. For example, if we say that how many chances are there that the president will remain the same this year? Now everyone would reply in percentage, like 15 percent chances or whatever.
Lorentz Distribution or Cauchy distribution is named after Augustin Cauchy. It has an undefined mean and variance. The two main parts of the Cauchy distribution tell us about where the peak is and the half-width of the graph.
We discussed Poisson distribution in the types of a discrete probability distribution. The time between the intervals of the Poisson experiment is known as an exponential or negative exponential distribution. It is used to test how reliable the product is.
Gamma Distribution has its applications in real life. We use it where the minimum value for the probability is zero. It has a mounded shape when the value is more significant than one. As the value increases, the shape becomes less skewed.
The logistic distribution has the same shape as the normal distribution and has only one peak. It is mainly used for logistic regression. Due to the exact shape of the logistic distribution and normal distribution, sometimes it becomes tough to identify one.
A Swedish mathematician Waloddi Weibull proposed Weibull Distribution, and it was named after him. Nowadays, Weibull distribution is mainly used for life data analysis and to assess the product’s reliability.
Frequently Asked Questions (FAQs)
How do you know if the probability distribution is discrete?
To check whether the probability is continuous or discrete, check out the two primary conditions. If the conditions apply, it means that the probability is discrete. Otherwise, the probability is continuous.
The first condition is that the probability must be between 1 and 0. If the probability is more or less than this limit, it means the probability is not discrete. Secondly, the sum of all the possible probabilities must be one. This is the second condition for checking the type of probability.
What is the discrete probability distribution? What are the two conditions?
A discrete probability distribution has a finite number of results. Like when we roll a dice, we can get one of the six sides. Now we have ⅙ chances that we can get any of the sides. This type of distribution with a finite number of results is known as a discrete probability distribution.
To check if the distribution probability is discrete or continuous, we have some conditions to apply. If the distribution is satisfying these two conditions, it means it is a discrete probability distribution.
The first condition is that the value of the discrete variable must be between zero and one. The second condition says that the sum of all the values of distribution must be equal to one. If any distribution satisfies these two conditions, it is a discrete probability distribution.
Does a discrete probability distribution have to equal one?
It is one of the two conditions that make the distribution a discrete probability distribution. If all the possible probabilities are not equal to one, then it is not a discrete probability distribution.
Final Words
In conclusion, we can say that probability distributions play many roles. They have an essential role in understanding and solving many statistical problems. Many subtypes of probability distributions are used in daily life. For example, when we check the probability of rolling dice or a tossed coin, distribution principles are used.
A discrete probability distribution is a statistical term that is essential if you are dealing with the probability topic. We discussed the types of probability distributions and then their subtypes briefly.
Both types of probability distribution have several subtypes in them, and also, the examples are included. For your convenience, we tried to write everything in simple and straightforward words. We would appreciate it if you ask us about your doubts in the comment section.