Table of Contents
Machine learning vs AI is the buzzword in the last few years. Both the jargon have become intrinsic to our daily lives. We are oblivious to the rapid impact of both domains.
Artificial intelligence encompasses training computers to demonstrate human-like behavior. Machine learning is a subdomain of artificial intelligence. It focuses on the automation of learning according to some performance metrics. Machine learning is heavily dependent on data to find hidden patterns.
A rapid rise in artificial intelligence domain has resulted in growing demand of business use cases. The whole world is shifting towards digitization. In this era need for developing and testing algorithms on data is more than ever. The importance of machine learning and the artificial intelligence domain has heightened.
What is Machine Learning?
The most exciting branch of Artificial intelligence, Machine learning has taken the world by storm. Machine learning accentuates the power of data in a whole new manner. The main agenda is to improve the customer experience.
There are plenty of daily life examples of machine learning. When you ask Alexa to search for top-rated movies or Cortana to play your favorite music. Machine learning algorithms skip other songs and play only those that were repetitively played by you.
Data is the food for machine learning. All the predictions are derived from data. So the more data we feed to the algorithms, the better results algorithms can derive. The applications designed from machine learning can derive computations from past data. Such applications have proven to be more reliable in pattern recognition and predictions. Machine learning applies different algorithms to data to bring out predictions.
There are plenty of famous and in-demand jobs in the machine learning industry. Some famous roles are:
- Machine learning engineer
- Machine learning developer
- Data scientist
- Computer vision engineer
For newbies, plenty of online and offline resources are available on the internet. You can earn certifications to further boost your portfolio.
Machine learning is dependent on three variables for better analysis and predictions. The three components are:
- Datasets (the bigger the better)
- Hardware i.e. improved and sophisticated
- Algorithms i.e. smart analysis
- Computer vision and natural language processing are two industries that have huge applications in machine learning.
Datasets
Training models are machine learning is reliant on dataset size. The more data we have, the better algorithms can make predictions. Accuracy will also be subsequently improved.
Hardware
With the gigantic increase in data, improved hardware is an imminent necessity for better results. For dealing with this much amount of data, models require extensive computation. Cost and time effectiveness is a supreme priority for better accuracy within less time.
Algorithms
Giants like Google and Amazon are focused on better algorithms. Because optimization is dependent on better and smarter algorithm implementations. Researchers are focused on improved versions of algorithms.
Required skills for machine learning
For learning machine learning there are a few requirements.
- One must have a basic knowledge of one of the programming languages i.e. Python, and R.
- Concepts of probability and statistics. An intermediate mathematical foundation is required for applying machine learning algorithms.
- The basic foundation of Linear algebra is a must. All the modeling and analysis require an understanding of linear algebra. Computer vision applications are dealt with matrices. Regression models are based on intersecting data through boundaries. All these computations are handled with linear algebra concepts.
- The basic understanding of calculus is also required. Differentiation concepts are frequently involved in machine learning algorithms.
Is it hard to learn machine learning?
Implementing algorithms and learning the specifics behind algorithms needs technical background as mentioned in the skill section above. It can take from 6 months to 18 months to become proficient in machine learning. This is subjective to the amount of time invested by the person. The type of projects and coursework chosen impacts the learning curve.
You can follow YouTube courses which are available for free. If you can afford to pay, join machine learning courses on famous platforms like Coursera, Udacity, Udemy. The most famous free course is by acclaimed Stanford professor Dr. Andrew Ng. it covers everything starting from the very basics to application building.
What is AI?
Artificial intelligence is impacting every walk of life. Artificial intelligence is altering every niche i.e. finance, security, smart cities, health care.
The impact of artificial intelligence on the business industry is astounding. The considerable potential for amplifying the profits is splendid.
When artificial intelligence is implemented in conjunction with machine learning, the results are marvelous.
Artificial intelligence has endless potential to bring revolutionary changes in every walk of life. There are different roles in the industry related to artificial intelligence. Some of them are AI architects, AI developers.
The most prominent industries to have applications in the artificial intelligence domain are as follows:
- Image and video recognition i.e. face recognition, photo tagging
- Video classification i.e. security cameras
- Recommendation systems i.e. product recommendations
- Time-series applications i.e. financial analysis
- Speech-to-text applications
Required skills for Artificial Intelligence
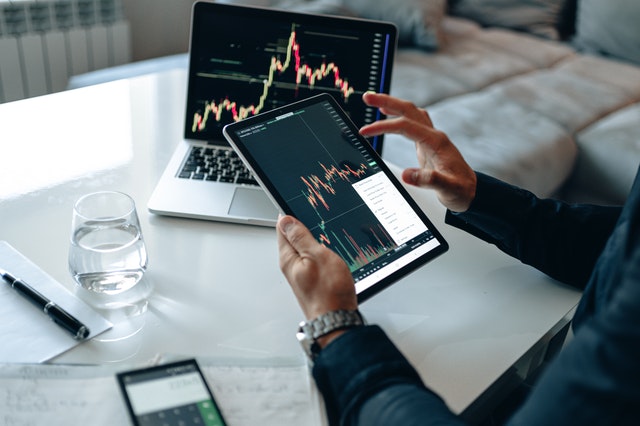
The skills required for an artificial intelligence engineer are as follows.
- You need to have a programming background. There is no restriction on language. Three main languages are used I this domain i.e. Python, R, Java. To develop and implement models, a good programming foundation can play a pivotal role.
- Linear algebra is the second requirement.
- A Mathematical foundation of statistics and probability is a bonus.
- Problem-solving skills are a must. The algorithms have to be applied to real-world problems. Good analytical skills can take you far ahead of the game.
Is it hard to learn artificial intelligence?
Learning artificial intelligence with the plethora of online and offline courses available is not much ado. The estimated time to become skilled depends on the time and schedule of the person. It can take from 6 months to 2 years to become proficient in this domain. If you are a newbie and have no prior experience, it can take more time.
Machine learning vs AI: key differences
There are certain key differences between artificial intelligence and machine learning.
- Artificial intelligence enables machines to simulate human-like behavior. Machine learning is a subdomain of artificial intelligence. Machine learning enables the machine to predict patterns based on past data.
- Artificial intelligence is more focused on solving complex problems by designing smart computer systems. On the other hand, machine learning emphasizes getting better output from past data. Accuracy of predictions is the major emphasis in machine learning.
- Artificial intelligence algorithms are designed to focus on reasoning and self-learning. In the machine learning domain, self-correction is highly dependent on new data.
- Artificial intelligence is subdivided into three categories i.e. Strong AI, Weak AI, artificial superintelligence. Machine learning has 3 subdomains i.e. Supervised learning, unsupervised learning, and reinforcement learning.
Categories of artificial intelligence
There are three broad categories of artificial intelligence.
Strong AI
Strong AI also known as general AI. It is a concept that implements general human intelligence and human behaviors. It is also known as Deep AI.
Weak AI
Weak AI is the subdomain that has seen the most success to date. Weak AI is goal-oriented. It is designed to focus on getting tasks done like speech recognition, self-driving cars.
Artificial Superintelligence
This domain aims to replicate human beings in terms of emotions as well as intelligence. Self-aware machines are included in this domain. The target is to make the machine super intelligent. Ultimate aim is to surpass the capabilities of human intelligence.
Future of AI and Machine Learning
The demand for technical professionals in this arena has quadrupled. The exponential growth in the applications in both domain has brought it to the frontier.
There is virtually no industry today that is not impacted by the revolutions brought by AI and machine learning. Transportation, health care, education, manufacturing, media, and customer service are some prominent domains highly impacted. The ramifications of both domains are astounding. Being mindful of the changes and adapting to these is the only key to success.
So, in terms of usage, there’s no above to one another. Please allow us to say that for machine learning vs AI — it’s a tie.